Report: Driving less won't hurt the economy
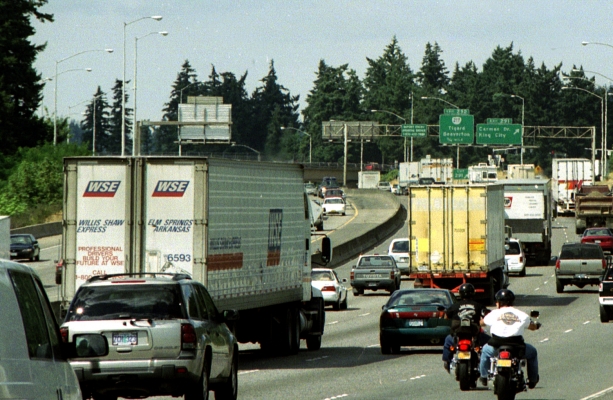
With various governments encouraging people to drive less, economists have wondered if such goals can have the side effect of harming the economy. In most cases, the answer is no, OTREC researcher B. Starr McMullen concluded in a research report.
- Click here to read more about the research and to download the report.
It’s more than an academic question: driving and the economy do tend to rise and fall together. McMullen, a transportation economics professor at Oregon State University, examined the relationship between the two by looking at which happens first—a change in driving or a change in economic activity.
In general, economic growth leads to more driving, not the other way around, McMullen said. That’s particularly true for metropolitan areas, the very places most likely to pursue policies that reduce driving.
“The more economic activity you have, the more VMT [vehicle miles traveled] you’re going to have,” McMullen said.
On the other hand, if there are policies to reduce VMT and driving decreases, “you’re not going to have the economy fall apart," as some have suggested.
If a state sets a goal to reduce VMT or transportation emissions, it is reasonable to expect big urban areas to enact policies first, as they have the most emissions and the worst congestion. “The place it’s most obvious to do it is the metropolitan level,” McMullen said. “This is where the debate is right now and where a lot of (transportation) policy is made.”
McMullen found that, in medium-sized or larger urban areas, there was no significant causal relationship between driving and economic activity in either direction. A few urban areas, including Portland, showed a two-way causal relationship between driving and economic growth.
However, when she controlled for factors that vary across urban areas—such as availability of transit, gas prices, population density, employment mix and the miles of road— this causal relationship was no longer observed in the Portland area. This demonstrates the need to look more closely at other factors that influence VMT and driving in each metropolitan area before trying to figure out the potential effects of VMT reduction policies.
Even using national data that included rural areas, the results show driving leading economic growth only when business cycles aren’t taken into account. Looking at data from 1929 to 2009, McMullen found that economic activity consistently preceded VMT, not the other way around.
In fact, the only periods in which VMT leads economic activity are economic downturns, and those downturns, like the Great Recession, have causes unrelated to driving. “In the business cycle downturn, VMT seems to strongly lead economic activity, but you can’t say that it’s the cause of the economic downturn,” McMullen said.
“We tested this on the business cycle and it clearly showed that VMT leads economic activity only in the downward side of the cycle and the rest of the time you have the expected causal relationship,” she said.
This report should encourage those who support policies to limit driving, although policy makers need to proceed with caution. Although McMullen looked at some factors that allow for mobility even as driving drops, each new policy warrants a thorough local examination.
“If you have a VMT reduction policy in an area with no transit and long commute distances, it’s bound to have more of an impact on people than an area with shorter distances and more transit or bicycling,” McMullen said. “You have to be careful to look at your own metropolitan area to see how your characteristics differ.”